Pro Tips
Nov 14, 2024
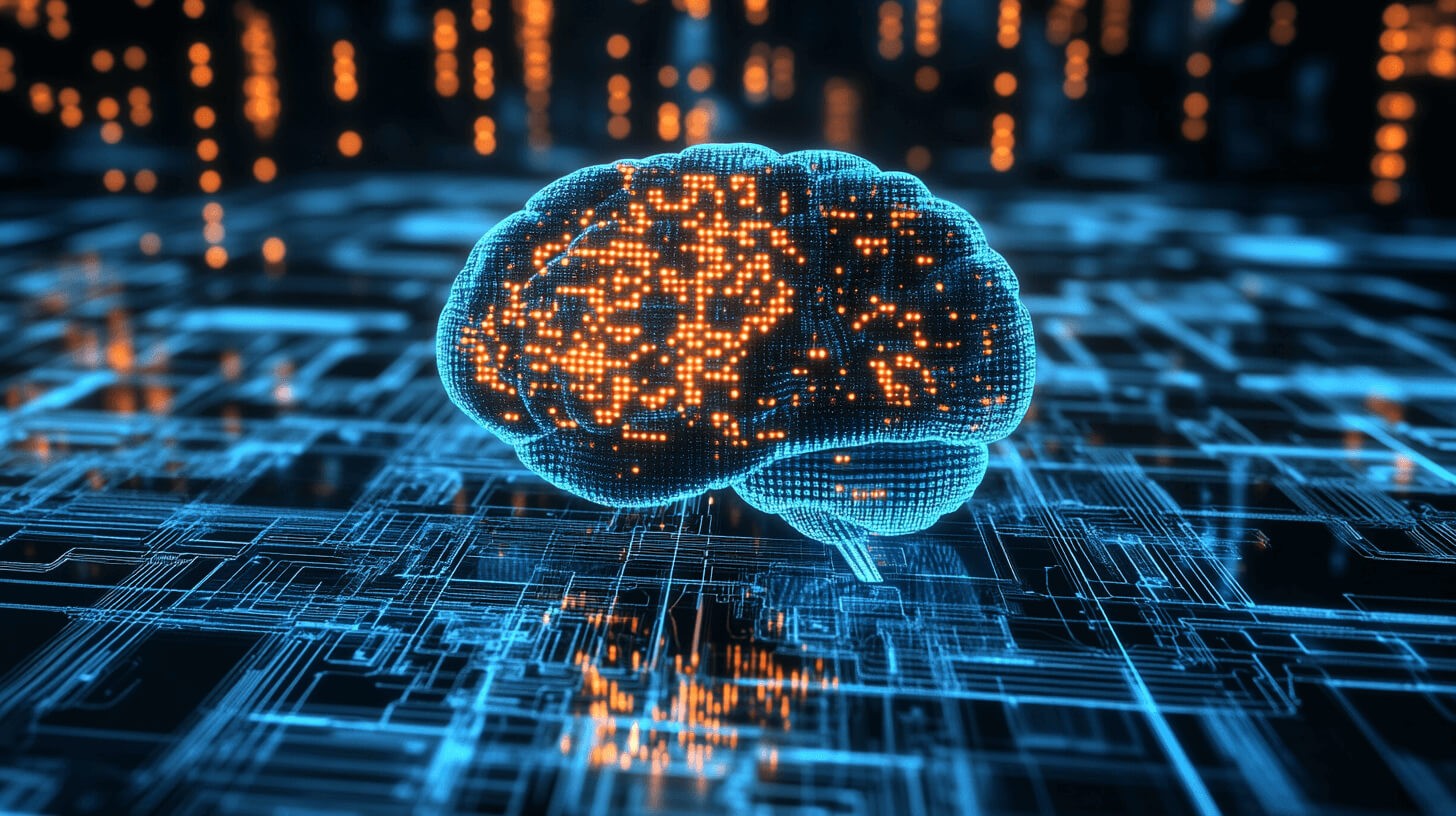
If you’ve ever found yourself searching endlessly for the right dataset, you’re not alone. Many professionals across various industries face this challenge. That’s where synthetic datasets come into play. These artificially generated datasets can be customized to meet specific requirements, providing solutions that traditional data sources often cannot.
What Are Synthetic Datasets?
Synthetic datasets are artificial data generated through algorithms rather than collected from real-world events. They mimic the statistical properties of real data while allowing for complete control over their structure and content. This capability is especially useful when working with sensitive information or when real data is scarce.
Benefits of Using Synthetic Data
One of the primary benefits of synthetic datasets is their flexibility. Users can define exactly what they need, whether it’s a simple list or a complex multi-column format. Additionally, generating synthetic data can save time and resources, eliminating the frustration associated with finding existing datasets. With platforms like Data Rush, users can quickly create, validate, and store these datasets without the hassle of traditional methods.
Use Cases Across Industries
Healthcare
In the healthcare sector, synthetic datasets are invaluable for research and development. Researchers can generate patient records that comply with privacy regulations, enabling them to perform analyses without compromising sensitive information. For example, a new drug trial may require diverse patient data, which can be synthesized to reflect various demographics and health conditions, ensuring comprehensive testing.
Finance
The finance industry heavily relies on data for decision-making processes. Synthetic datasets can simulate market conditions, helping analysts test financial models without risking actual capital. Imagine a bank wanting to evaluate its risk management strategies; it can use synthetic data to model different economic scenarios and assess potential outcomes without exposing itself to real-world volatility.
Machine Learning
Machine learning practitioners often require large amounts of high-quality training data. Synthetic datasets can bridge this gap by providing tailored data that meets specific algorithmic needs. For instance, if a developer is building a natural language processing model, they can create datasets with various linguistic structures to improve the model's accuracy. Tools like Data Rush allow users to customize their datasets effectively, enhancing the quality of their machine learning projects.
How Data Rush Facilitates Dataset Creation
Data Rush stands out as a user-friendly platform that simplifies the process of synthetic dataset creation. Users can specify the number of columns and types of content, ensuring that the final product aligns perfectly with their project requirements. The platform also offers advanced functionalities such as custom validations and filtering options, making it easier to refine datasets according to specific rules.
Moreover, the sampling feature lets users preview a portion of their dataset before finalizing it, ensuring satisfaction with the output. This level of customization and flexibility makes Data Rush an excellent choice for anyone looking to leverage synthetic data.
Conclusion
As we've seen, synthetic datasets offer immense potential across various industries, from healthcare to finance and machine learning. They provide tailored solutions that address common data challenges, enabling organizations to make informed decisions without the constraints of traditional data sourcing. If you’re considering exploring synthetic datasets, platforms like Data Rush can help streamline your data generation process, ultimately saving you time and resources.