CMS
Basics
Nov 9, 2024
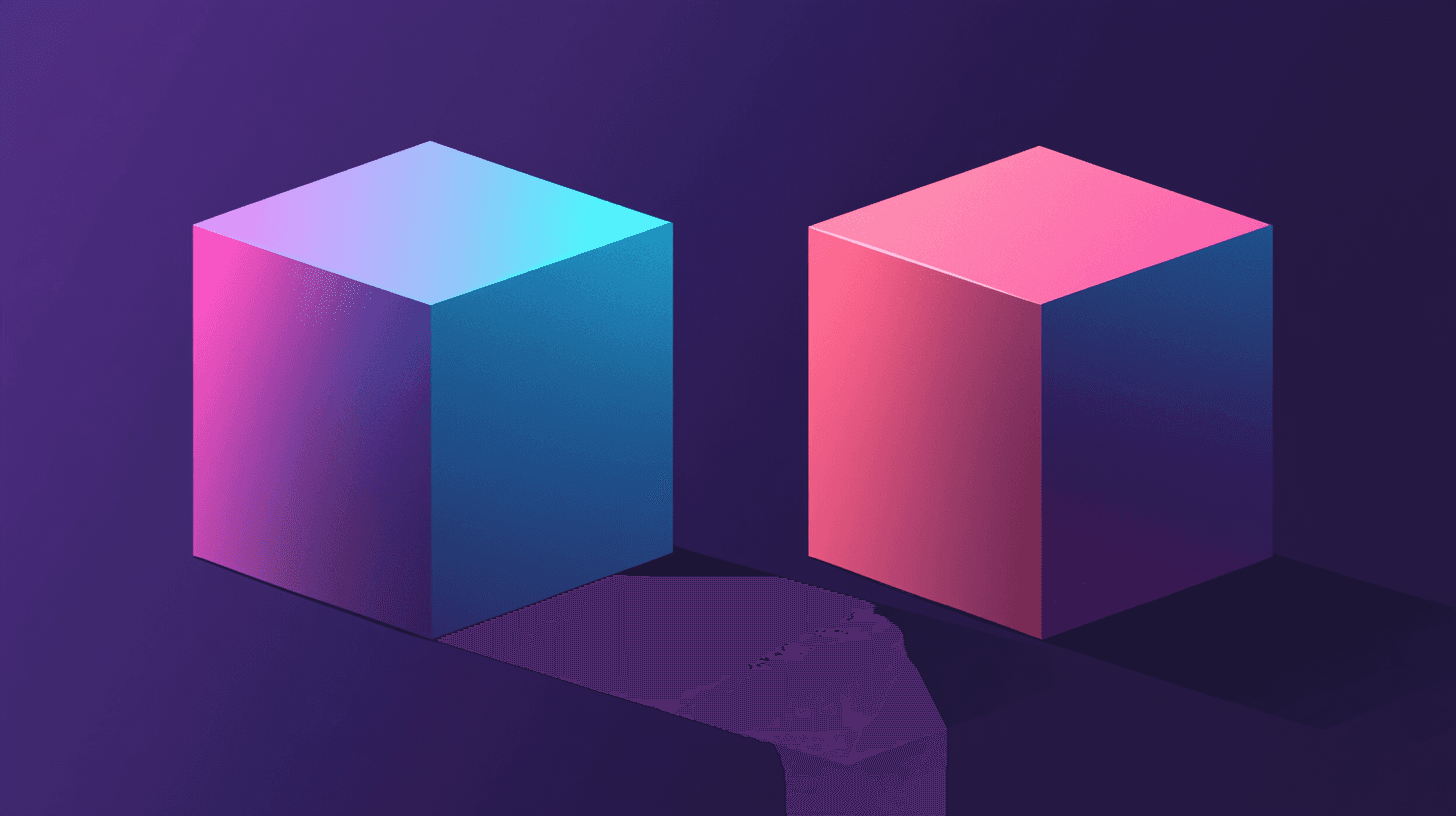
When it comes to data-driven decision making, understanding the differences between synthetic data and real data is crucial. If you've ever found yourself grappling with questions about which type of data to use for your projects, you are not alone. This guide aims to clarify the pros and cons of synthetic versus real data, helping you make informed decisions that can save time and resources.
What is Synthetic Data?
Synthetic data is artificially generated information that mimics the properties of real data without containing any actual personal or sensitive information. It's created using algorithms and can be tailored to meet specific criteria, making it a versatile choice for many applications.
Advantages of Synthetic Data
Cost-Efficiency: Generating synthetic data can often be less expensive than collecting and processing real data, especially when large volumes are required.
Privacy Assurance: Since synthetic data does not reference real individuals or entities, it significantly reduces the risk of privacy violations. This is particularly valuable for industries dealing with sensitive information.
Flexibility and Speed: Platforms like Data Rush allow users to create custom datasets rapidly. You can specify the structure and validation criteria, which means you can prototype and test solutions within minutes.
What is Real Data?
Real data, conversely, is information collected from natural sources, like user interactions or transaction records. While it offers unique insights, it also has its own set of challenges.
Advantages of Real Data
Authenticity: Real data reflects actual behaviors and transactions, making it perfect for analyses that require a genuine context.
Rich Insights: Since it comes directly from users, real data often provides better insights into customer preferences and market trends.
Key Differences Between Synthetic Data and Real Data
Cost
Synthetic data typically comes at a lower cost, helping organizations reduce expenses on data collection and processing. In contrast, real data often requires significant investment in gathering and maintaining.
Privacy Concerns
The usage of real data necessitates stringent compliance with data protection regulations, while synthetic data eliminates these concerns, making it easier to innovate without legal implications.
Volume and Variety
Synthetic data can be produced in limitless volumes, tailored to specific needs, which allows quicker scalability. Real data is limited by the sources available to you and can take more time to gather and analyze.
Use Cases for Each Type of Data
Where Synthetic Data Excels
Software Testing: Developers can utilize synthetic data to run tests without worrying about privacy breaches or data scarcity.
Machine Learning: In training models, synthetic data can provide the breadth and depth needed for effective learning outcomes.
Real Data Scenarios
Market Research: Analyzing consumer patterns and feedback using real data can yield invaluable insights that guide strategy.
Defining Customer Segments: Understanding the nuances of customer behavior often requires real data for accurate segmentation.
Making the Right Choice
Choosing between synthetic and real data is not always straightforward. It often depends on your specific project needs. If you're looking for rapid development with privacy at the forefront, synthetic data is ideal. However, for in-depth market analysis and authentic insights, real data is indispensable.
Conclusion
Both synthetic and real data have their strengths and weaknesses. As technology continues to advance, the importance of understanding these two data types becomes even more critical. By leveraging platforms like Data Rush, you can create and work with custom datasets that suit your precise needs, empowering your data strategy for success.